Bits of advice: the true colours of dark patterns
Dark patterns are deceptive online interface designs that are used to trick people into making decisions that are in the interests of the online business, but at the expense of the user. In this second article of our ‘bits of advice’ series on digital regulation, we dive into the economics behind dark patterns: what are they; what can economics teach us about how they work; how is digitalisation changing their costs and benefits in the eyes of businesses; and how can competition and consumer protection authorities respond?
We are grateful for valuable input from and discussions with Dries Cuijpers and Annemieke Tuinstra of the Netherlands Authority for Consumers and Markets (Autoriteit Consument en Markt, ACM) in writing this article.1
On 28 October 2021, the US Federal Trade Commission (FTC) announced its plans to increase enforcement against ‘illegal dark patterns’ that mislead consumers into buying subscriptions.2 This policy statement comes shortly after the FTC announced that curbing ‘deceptive and manipulative conduct on the internet’ was to be one of its eight enforcement priority areas over the next ten years.3
In Europe, authorities have identified the online world as an area of attention in terms of consumer protection. For example, in February 2020 the Netherlands Authority for Consumers and Markets (Autoriteit Consument en Markt, ACM) published its guidelines for the protection of online consumers,4 and in July 2020 the UK Competition and Markets Authority (CMA) completed its study into the role of ‘choice architecture’ in inhibiting effective consumer decision-making on online platforms and in digital advertising.5
Much of the discussion around deceptive and manipulative online conduct revolves around ‘dark patterns’—which we define simply as deceptive online interface designs that are used to trick people into making decisions that are in the interests of the online business, but at the expense of the user.6 These designs may pertain to websites, but also to games or apps.
Recognised examples of dark patterns include:7
- excessive urgency messaging—where an online retailer creates a (possibly false) sense of scarcity that urges consumers to buy immediately;
- drip pricing—additional surcharges that become clear only once a consumer is about to pay for the selected product;
- bait-and-switch—where an online retailer lures users onto its website with unique, high-quality, or cheap products that the business knows are not actually in stock, in order to sell alternatives;
- disguised advertisements—for example, a social media advertisement disguised as a regular social media post;
- subscription traps—for example, an enticing free trial subscription that then automatically continues into a paid subscription that is complicated to cancel;
- misdirection—the use of visuals or language to steer users towards a particular choice;
- exploitative default settings—misdirection in which consumers consent to certain choices (such as privacy-intrusive settings) because of unclear or burdensome default settings;
- confirmshaming—misdirection in which users are ‘guilted’ into opting into something—for example, by wording the option to decline in such a way that it shames the user.
Figure 1 provides a stylised illustration of different types of dark pattern.
Figure 1 Illustrative dark patterns
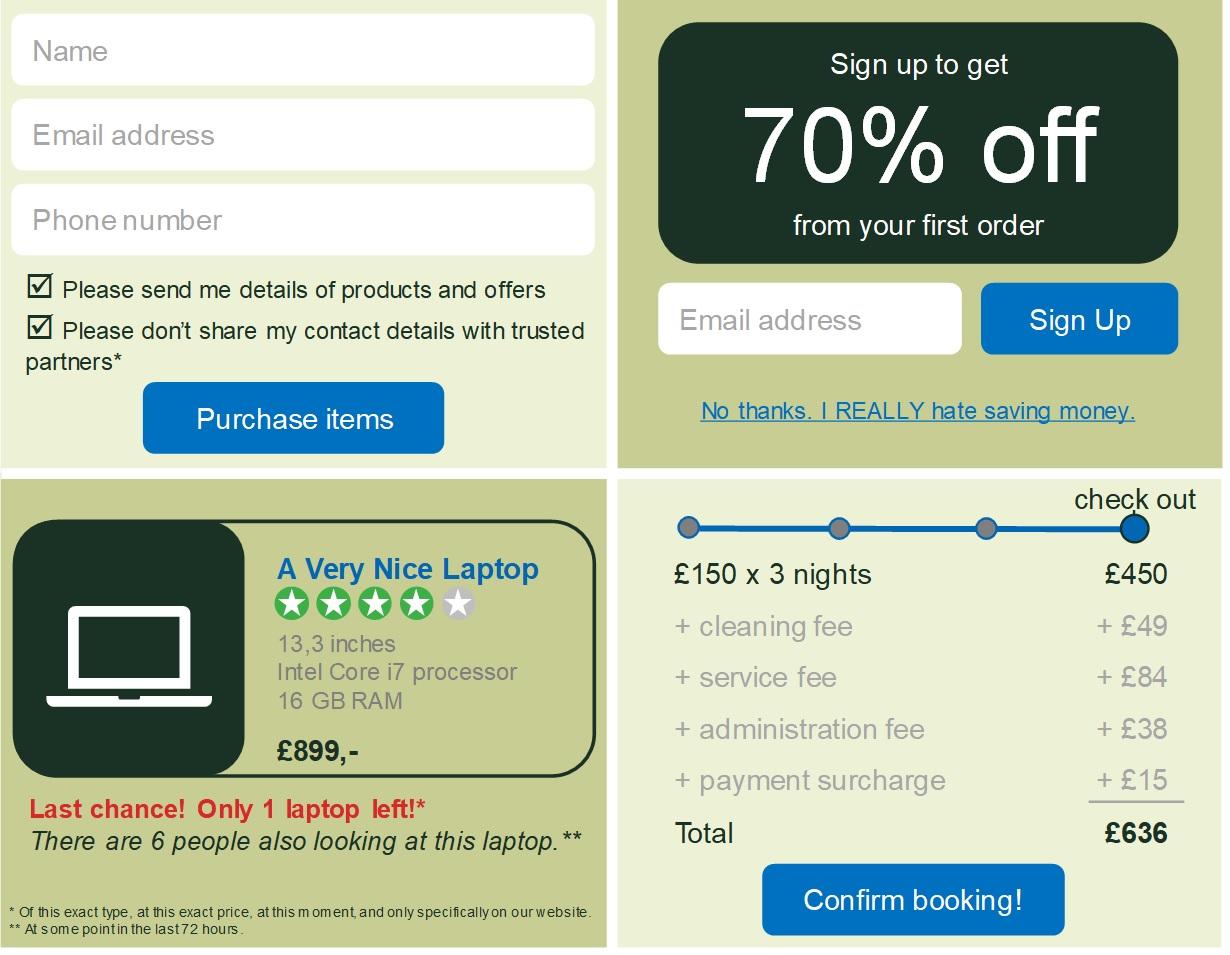
Source: Oxera.
But what do we actually know of the economics behind dark patterns?
This article considers ‘the old’ and ‘the new’ economics behind dark patterns. In particular, we discuss how behavioural economics can help us to understand the workings of dark patterns, and how digitalisation has changed the costs and benefits behind their implementation and exploitation. We end with a discussion of how economics can inform ongoing policy discussions on appropriate regulatory responses, and how it can help to assess the actual effects of the alleged use of dark patterns.
What’s old: the behavioural economics behind dark patterns
Traditional economics assumes that, as humans, we use all available information before us and process this in a purely rational way in order to make optimal decisions. However, in reality there are limits to our ability to do this. Behavioural economics increases the explanatory power of economics by providing it with more realistic psychological foundations.8
To provide a more accurate description of human problem-solving capabilities, behavioural economists coined the term ‘bounded rationality’. Since an individual’s brainpower and time are limited, humans cannot be expected to solve difficult problems ‘optimally’; rather, people adopt rules of thumb as a way to use their effort and time efficiently.9 However, such ‘heuristics’ (or even relying on pure instinct) can lead to systematic and predictable errors in situations of uncertainty.10 These errors can also be referred to as behavioural (or cognitive) biases.11
Behavioural economics tells us that—because of these biases—the way in which information or choices are presented can have a significant impact on the decisions that individuals make.12 This is discussed in more detail in the box below.
Cognitive biases
Behavioural economists have identified a wide range of biases. Some of the most relevant in the context of dark patterns are as follows.
- Default bias is the tendency of people to disproportionately stick with the status quo. For example, subjects in experimental studies are frequently found to stick to default choices more frequently than would be predicted by standard economics.1 The effect is visible in many important decisions—for example, in the selection of health plans and retirement options.
- Scarcity bias is the tendency of people to place a higher value on things that seem scarce.2 Some websites make use of this bias by displaying countdown timers or limited-time messages to create an excessive sense of urgency.
- The social norm effect is the tendency of people to value something more because others seem to value it—or, more generally, to simply follow the crowd.3 For example, individuals are more likely to impulse buy if they are shopping with their peers and families than if they are shopping alone.4 Websites that use excessive urgency messaging based on the activity of other users, use disguised social media advertisements, or deploy confirmshaming, may rely on this effect.
- Loss aversion is the tendency of people to increase the relevance of (potential) losses in their decision-making relative to corresponding gains. This discrepancy has been labelled the endowment effect, because the value that people associate with something appears to change when it is included in one’s endowment.5 The loss aversion mechanism may play a role in (for example) drip pricing or subscription traps.
Note: 1 See, for example, Samuelson, W. and Zeckhauser, R. (1988), ‘Status quo bias in decision making’, Journal of Risk and Uncertainty, 1:1, pp. 7–59 for an early account of this. 2 Mittone, L. and Savadori, L. (2009), ‘The scarcity bias’, Applied Psychology, 58:3, pp. 453–68. 3 Sherif, M. (1936), The Psychology of Social Norms, Harper. 4 Luo, X. (2005), ‘How does shopping with others influence impulsive purchasing?’, Journal of Consumer Psychology, 15:4, pp. 288–94. 5 Tversky, A. and Kahneman, D. (1991), ‘Loss aversion in riskless choice: A reference-dependent model’, The Quarterly Journal of Economics, 106:4, pp. 1039–61; and Thaler, R. (1980), ‘Toward a positive theory of consumer choice’, Journal of Economic Behavior & Organization, 1:1, pp. 39–60.
Insights from behavioural economics can be used to support benevolent policy objectives: a policymaker can design ‘choice architectures’ such that people are promoted to make better decisions, without impeding on their freedom to choose.
For example, healthy food options can be displayed more prominently within a canteen or supermarket in order to promote a healthier lifestyle. This is often referred to as ‘nudging’, which was popularised by Richard Thaler and Cass Sunstein in their 2008 book Nudge: Improving Decisions about Health, Wealth, and Happiness. Thaler received the 2017 Nobel Prize in Economic Sciences for his contributions to behavioural economics.13
However, insights into behavioural economics are not only or always used for good. More recently, Thaler coined the term ‘sludge’ to refer to all activities ‘that are essentially nudging for evil’.14
For example, any type of subscription that is easy to sign up for but incredibly difficult to cancel can be considered a sludge. Governments can implement sludges—for example, by making it more difficult for people to register to vote. The only practical difference between a sludge and a dark pattern therefore seems to be that a sludge is a concept that can be applied much more broadly to both offline and online practices, whereas the concept of dark patterns is more specifically applied to online practices.15
As such, the link between the established literature on behavioural economics and dark patterns does raise another question: what’s new? Is this discussion around dark patterns not simply old wine in new bottles?
What’s new: digitalisation and ‘hypernudging’
In recent years dark patterns have become much more pervasive, due to two key developments in the digital space.16
Minimal costs
First, the costs of experimenting with different user interface designs have decreased tremendously. In a digital environment, businesses are able to experiment effectively with different website designs through A|B testing (where some users are shown a different layout to others) and investigate the effects at little cost. Cheap A|B testing can help webpage developers to quickly identify ways to incrementally improve the design of a webpage—to the benefit of users—or to exploit biases and change user behaviour for the benefit of the designer.
Vast gains
Second, the scale of potential gains has vastly increased as a direct result of increased digital distribution and globalisation. With many online businesses operating at a large scale, even minor changes to a user interface can have huge benefits for the business in absolute terms.
To see the relevance of both lower costs and higher gains in the digital world, contrast this with a physical grocery store changing the layout of its shelves to entice customers to buy its more profitable products—for example, by placing them in more prominent areas.17 The owner is in principle able to experiment with different layouts. However, implementing such an experiment offline is much less practical than it is online: it takes a significant amount of time to set up different variants of the shelves and to expose these to a sufficient number of (ideally randomised) shoppers. Moreover, once a more profitable layout has been identified, the grocery owner is able to roll out this more profitable layout only across its own stores.
In contrast, an online business can readily implement and experiment with different webpage designs, assigning users randomly to each design and retaining those designs that show better performance. There is relatively little cost to changing the choice architecture. Moreover, if the online business is operating globally, even minor improvements can immediately be implemented at scale.
This has even raised concerns of ‘hypernudging’, where the online choice environment for consumers is adapted in real time (and potentially even personalised to individual consumers) in order to stimulate more sales, based on extensive data collection on online consumer behaviour.18 While offline shops can display their products in one only way, online shops could condition this on customer-specific behavioural clues.19
What to do: competition policy and regulation
As concerns around dark patterns increase, policymakers, regulators and authorities are faced with the complex challenge of determining what role they should play. For example, can we trust the competitive process to ‘compete away’ undesirable dark patterns, or is intervention or self-regulation required? And if so, in what form?
Why competition policy is not enough
Deceiving users can be bad for business: in cases where there is strong competition, the threat of customer switching may pressure firms to abstain from (at least) the most aggressive forms of dark patterns. Research has shown that—at least in some cases—users punish the most aggressive forms of dark patterns if they have other options available.20 This also means that dark patterns may be exceptionally pervasive when firms have more market power: when competition is limited, users are less able to switch away in the first place, enabling businesses to pursue aggressive strategies.21 Protecting the competitive process may then help to reduce the risk of exploitative abuses in the form of dark patterns.
However, this competition mechanism may not be strong enough. For example, competition may not prevent firms from deploying more subtle forms of dark patterns, especially when consumers are uninformed or in some way vulnerable.
Moreover, the costs and benefits involved in deploying dark patterns may change under increased competition—incentivising firms to deploy more, rather than fewer, dark patterns. For instance, theoretical behavioural economics research has shown that, in the presence of consumer biases, firms may be better off increasing certain forms of behavioural exploitation—such as drip pricing—under increased competition.22 Nobel Prize laureates George Akerlof and Robert Shiller even claim more generally that competition can pressure firms to ‘phish for phools’ (i.e. exploit human behavioural weaknesses), because if they do not, they will be replaced by competitors that will.23
Regulation and consumer protection
In short, a healthy competitive process helps to reduce the risk of some exploitative dark patterns, but consumer protection law may also have a substantial role to play. Indeed, consumer protection law more generally recognises that markets cannot solve all forms of consumer exploitation.
It is therefore no surprise that many consumer protection authorities have already engaged actively with this topic—including the CMA, the ACM, the FTC, and the Norwegian Consumer Council.24 For example, in February 2020 the ACM published its guidelines for the protection of online consumers.25 Such guidelines can provide clarity for firms on what is expected of them.
At the same time, guidelines can quickly become outdated in sectors that see rapid developments. For that reason, the ACM already has a revision of its guidelines in the works (although a projected date is yet to be announced).
Setting rules or setting goals?
Whether the increased enforcement of current competition and consumer protection law is sufficient to address the problem of dark patterns remains to be seen.
Protecting the competitive process will surely help, as will imposing consumer protection rules on digital businesses to specify what they may or may not do. However, ruling on which procedures should be followed may not always work—particularly when a sector is as fast-paced and dynamic as the digital sector.
As discussed in the previous Agenda article in this series, goals-based regulation—where objectives are specified, but the process for how to reach them is not—may then have advantages.26 At the same time, setting general goals rather than precise rules may create uncertainty and reduce clarity in the market.
Ideally, therefore, a mix of both rules-based and goals-based regulation may be best.
One way in which rules-based and goals-based regulation can be combined is through the use of safe harbours. One example of this in the context of dark patterns is the 2019 Deceptive Experiences to Online Users Reduction (DETOUR) Act in the USA. The Act aims to reduce dark patterns by making it unlawful to design a user interface with the intention or effectof impairing user autonomy or decision-making.27 It employs safe harbours for services with fewer than 100m monthly users,28 or services that establish default settings that provide enhanced privacy protections to users.
Assessing effects
Finally, it should be noted that there may be a substantial risk to innovation and product improvement if regulators and competition authorities take a dogmatic approach to dark patterns and behavioural influencing more generally.
Not all behavioural influencing is harmful to consumers. In cases of uncertainty, economic effects analysis is required to determine whether the issues are truly harmful to competition and users—as the DETOUR Act recognises.
This brings us to the next step in the debate on dark patterns: how to assess their actual effects or damages. Assessing effects can benefit all parties involved. In particular, it can:
- support regulatory authorities in the prioritisation of their work;
- support firms in identifying exploitative practices and managing their regulatory risk;
- support users and firms in estimating the appropriate amount of compensation where there is a dispute about the harm suffered.
Although much more research and thinking is required in this area, there are several promising avenues.
First, the results of online A|B tests could be subjected to regulatory scrutiny, or new A|B tests could be implemented to identify the net effects of different user interface designs on users—from the perspective of the user’s best interests rather than the firm’s.
Second, key consumer metrics in the presence of dark patterns could be compared across groups, across time, or through ‘difference-in-differences’ tests. Such comparator-based analyses are already standard in the context of antitrust damages,29 and there is no reason in principle why they cannot also be used in the context of online abusive designs.
As the development of these tools is likely to keep pace with the advent of dark patterns, it is bound to be an exciting area of future research.
1 Any remaining errors are our own. In the first article of this series, we discussed how the principles behind rules- and goals-based regulation can inform the regulatory debate on data access. See Oxera (2021), ‘Bits of advice: how to regulate data access’, Agenda, September.
2 Federal Trade Commission (2021), ‘FTC to ramp up enforcement against illegal dark patterns that trick or trap consumers into subscriptions’, 28 October.
3 See Federal Trade Commission (2021), ‘FTC streamlines consumer protection and competition investigations in eight key enforcement areas to enable higher caseload’, 14 September.
4 Netherlands Authority for Consumers and Markets (2020), ‘ACM guidelines on the protection of the online consumer – boundaries of online persuasion’.
5 Competition and Markets Authority (2020), ‘Online platforms and digital advertising: market study final report’, 1 July.
6 This term was originally coined in 2010 by Harry Brignull, a user experience specialist, who defined dark patterns as ‘tricks used in websites and apps that make you do things that you didn’t mean to, like buying or signing up for something’ (see www.darkpatterns.org). Another common definition is that of user interfaces that lead consumers into making decisions that benefit the online business, but that the users would not have made if they were fully informed and capable of selecting alternatives. See Mathur, A., Acar, G., Friedman, M.J., Lucherini, E., Mayer, J., Chetty, M. Narayanan, A. (2019), ‘Dark patterns at scale: findings from a crawl of 11K shopping websites’, Proceedings of the ACM on Human-Computer Interaction, 3:CSCW, pp. 1–32.
7 See www.darkpatterns.org and OECD (2021), ‘Roundtable on dark commercial patterns online: summary of discussion’, 19 February.
8 Early advances in behavioural economics were made particularly by Daniel Kahneman and Amos Tversky—see, in particular, Kahneman, D. and Tversky, A. (1979), ‘Prospect theory: an analysis of decision under risk’, Econometrica, 47:2, pp. 263–92; and Tversky, A. and Kahneman, D. (1992), ‘Advances in prospect theory: cumulative representation of uncertainty’, Journal of Risk and Uncertainty, 5:4, pp. 297–323. Kahneman even received the Nobel Prize in Economic Sciences in 2002 for ‘having integrated insights from psychological research into economic science, especially concerning human judgment and decision-making under uncertainty’. It is widely considered that Tversky would have won this prize jointly with Kahneman, had he not passed away several years before. In 2011, Kahneman published the bestselling book Thinking, Fast and Slow, in which he summarises much of his research—see Kahneman, D. (2011), Thinking, fast and slow, Farrar, Straus and Giroux.
9 Simon, H.A. (1955), ‘A behavioral model of rational choice’, The Quarterly Journal of Economics, 69:1, pp. 99–118. Conlisk, J. (1996), ‘Why bounded rationality?’, Journal of Economic Literature, 34:2, pp. 669–700.
10 For an early account for this see, in particular, Tversky, A. and Kahneman, D. (1974), ‘Judgment under uncertainty: heuristics and biases’, Science, 185:415, pp. 1124–31. See also Kahneman, D. (2011), Thinking, fast and slow, Farrar, Straus and Giroux.
11 We omit here a discussion on whether cognitive biases may be caused by irrationality (i.e. inconsistency in revealed preferences), or whether they are the result of a rational response to cognitive costs (i.e. the mental efforts involved in making decisions).
12 This is also discussed in Oxera (2021), ‘Danger mouse: the opportunities and risks of digital distribution’, Agenda, April.
13 Thaler, R.H. and Sunstein, C.R. (2008), Nudge: Improving Decisions About Health, Wealth, and Happiness, Yale University Press; and Thaler, R.H. and Sunstein, C.R. (2021), Nudge: The Final Edition, Penguin Books. See also Oxera (2017), ‘The science of misbehaving: Richard Thaler wins the Nobel Prize’, Agenda, October.
14 Thaler, R.H. (2018), ‘Nudge, not sludge’, Science, 361:6401, p. 431.
15 On the link between nudging and dark patterns, see also Waldman, A.E. (2020), ‘Cognitive biases, dark patterns, and the “privacy paradox”, Current Opinion in Psychology, 31, pp. 105–9; and Bösch, C., Erb, B., Kargl, F., Kopp, H. and Pfattheicher, S. (2016), ‘Tales from the dark side: privacy dark strategies and privacy dark patterns’, Proceedings on Privacy Enhancing Technologies, 2016:4, pp. 237–54.
16 See also OECD (2021), ‘Roundtable on Dark Commercial Patterns Online: Summary of discussion’, 19 February. In addition to the reasons outlined, concerns have been raised about dark patterns that take advantage of biases and preferences at the level of individual consumers based on their data and previous usage patterns. See, for instance, Stigler Center (2019), ‘Stigler committee on digital platforms: final report’, September. Privacy law may protect consumers to some extent in such cases.
17 Were the owner of the grocery store to take a more paternalistic approach (i.e. more in line with nudging, as discussed in the box above), they might choose to place the healthier products on the most prominent shelves instead.
18 Yeung, K. (2017), ‘“Hypernudge”: Big data as a mode of regulation by design’, Information, Communication & Society, 20:1, pp. 118–36; and Oxera (2020), ‘Consumer protection in the online economy’, Agenda, March.
19 A discussion on this point in the context of sponsored ranking is provided by the ACM in Authority for Consumers and Markets (2021), ‘Sponsored Ranking: an exploration of its effects on consumer welfare’, 2 February, para. 64: ‘While this may serve consumers in the sense that it facilitates their search, it also means that individual consumers’ consideration sets, i.e. the set of products they compare out of the vast amount of products offered on the platform, can be tailored to extract their maximum willingness to pay. Possibly even more than that, if they perceive the sponsored product to be better than it actually is because it is higher up in the ranking’.
20 Luguri, J. and Strahilevitz, L.J. (2021), ‘Shining a light on dark patterns’, Journal of Legal Analysis, 13:1, pp. 43–109.
21 Stigler Center (2019), ‘Stigler committee on digital platforms: final report’, September. Day, G. and Stemler, A. (2020), ‘Are dark patterns anticompetitive?’, Alabama Law Review, 72:1, pp. 1–45.
22 Gabaix, X. and Laibson, D. (2006), ‘Shrouded attributes, consumer myopia, and information suppression in competitive markets’, The Quarterly Journal of Economics, 121:2, pp. 505–40.
23 Akerlof, G.A. and Shiller, R.J. (2015) Phishing for Phools: The Economics of Manipulation and Deception, Princeton University Press. A broader discussion on this topic is provided, for example, by Tuinstra, A., Onderstal, S. and Potters, J. (2020), ‘Experimenten voor mededigingsbeleid’, KVS Preadviezen 2020 (in Dutch).
24 Competition and Markets Authority (2019), ‘Online hotel booking’; Authority for Consumers and Markets (2020), ‘ACM guidelines on the protection of the online consumer – boundaries of online persuasion’; Forbrukerradet (2020), ‘Out of control: how consumers are exploited by the online advertising industry’, 14 January; Forbrukerradet (2018), ‘Every step you take: how deceptive design lets Google track users 24/7’, 27 November; Forbrukerradet (2018), ‘Deceived by design: how tech companies use dark patterns to discourage us from exercising our rights to privacy’, 27 June; OECD (2021), ‘Roundtable on dark commercial patterns online: summary of discussion’, 19 February; Federal Trade Commission (2021), ‘FTC streamlines consumer protection and competition investigations in eight key enforcement areas to enable higher caseload’, 14 September.
25 Authority for Consumers and Markets (2020), ‘Protection of the online consumer: boundaries of online persuasion’, February. See also Oxera (2020), ‘Consumer protection in the online economy’, Agenda, March.
26 See Oxera (2021), ‘Bits of advice: how to regulate data access’, Agenda, September.
27 DETOUR Act, S. 1084, 116th Congress, (2020).
28 However, the absolute size of a firm may not imply a concern about competition or consumer protection.
29 Oxera (2009), ‘Quantifying antitrust damages: towards non-binding guidance for courts’, study prepared for the European Commission, December.
Related
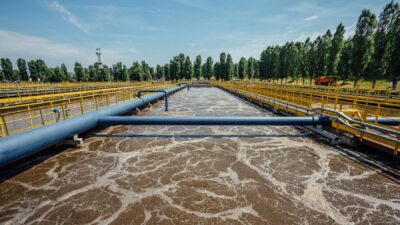
The future funding of the England & Wales water sector: Ofwat’s draft determinations
On Thursday 11 July, Ofwat (the England and Wales water regulator) published its much anticipated Draft Determinations (DDs). As part of the PR24 price review, this sets out its provisional assessment of allowed revenues and performance targets for AMP8 (2025–30)—and will be of great interest to water companies, investors,… Read More
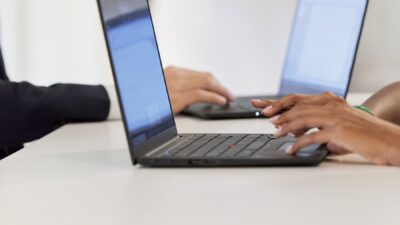
Deceptive patterns: how can we make choices fairer online?
Online, every click and swipe is subtly influenced by how choices are presented to you. In a world where what we watch, listen to, read, and buy is the result of responding to the choices presented to us on a screen, this choice architecture is hugely important. We may not… Read More