Agents of change: bringing economic models closer to reality
Ever wondered how economic models can describe people and their behaviour? Agent-based modelling (ABM) may be the answer. It allows economists to explore complex systems and phenomena in a bottom-up, data-driven way. Unlike traditional economic models, which are often constrained by assumptions and simplifications, ABM offers a flexible and powerful way to study the behaviour of individuals and groups in dynamic environments.
Economics can help us to understand how a particular industry works, the interactions between firms, the effects of a certain policy, or how some individuals’ actions might affect others. A variety of theoretical and empirical methods are available that allow us to compare the paths not taken (known as counterfactuals) with the actual path that led to an observed outcome.
When trying to understand, for example, the impacts of a proposed government policy, or the drivers causing a spike in pricing by a monopolist, the possibility of being able to observe the mechanisms that lead to specific results is of particular interest. This is known as the tractability of a model. But making a model tractable often requires trade-offs: simple models are more tractable, but require assumptions that are often not realistic. However, complex models can be criticised as being ‘black boxes’, where the link between inputs and outputs is difficult or impossible to understand.
With the rise of computational power and ever-larger datasets, new tools have started to be used by practitioners and academics. One of these tools promises to offer a balance between complexity and tractability. Its supporters argue that it allows for the exploration of complex systems without compromising the interpretation of results. In the scientific literature, the technique is commonly referred to as agent-based modelling (ABM).
What is agent-based modelling?
Imagine an island with one dictator and four people (Figure 1 below). The island is full of stone and wood, which can be collected, traded and used to build houses. The dictator periodically taxes people’s income, which is generated by trading resources or building houses.1 An ABM simulation would allow us to answer, for example: what are the resources left on the island at the end of the simulation? What taxes have been collected? How many houses have been built? We would be able to observe these metrics in every period during the simulation. Additionally, we would be able to select different starting points, different behaviours of the people living on the island, or different rules imposed by the dictator.
Figure 1 The dictator’s island
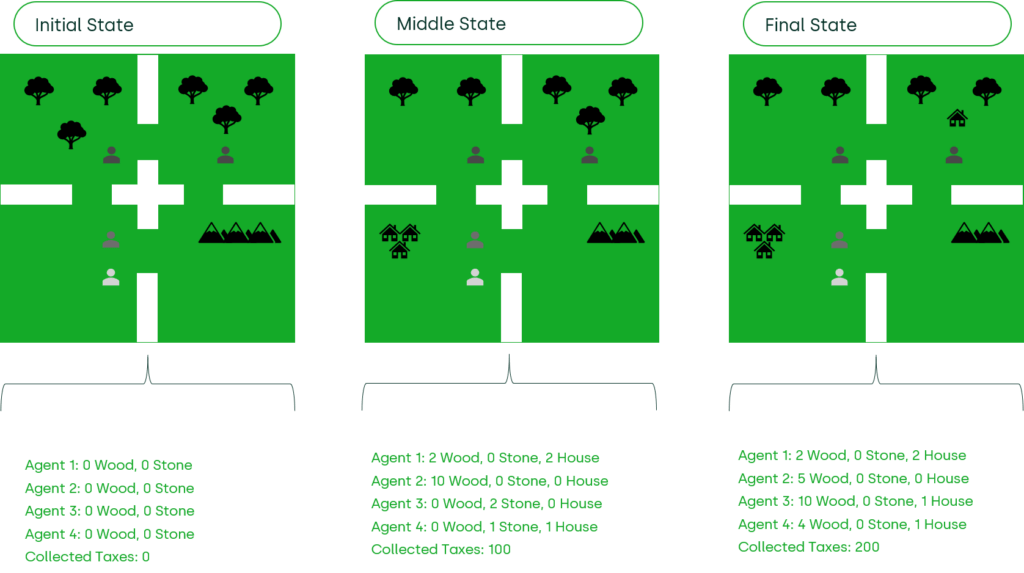
Source: Oxera, based on the example in Zheng, S., Trott, A., Srinivasa, S., Naik, N., Gruesbeck, M., Parkes, D.C. and Socher, R. (2020), ‘The AI Economist: Improving Equality and Productivity with AI-Driven Tax Policies’, arXiv preprint arXiv:2004.13332.
ABM is a technique to simulate scenarios that involve complex behaviour. It uses a bottom-up approach, leveraging computational power and programming languages to simulate agent behaviour, and observing the reactions of individuals to different inputs. It has several applications, ranging from fluid flow simulation in engineering, to market simulation in economics.2 ABM has the potential to change how we analyse complex systems.3 Due to its focus on building bottom-up simulations, it can describe and analyse dynamics that are beyond the reach of other analytical methods.
ABM is not based on a single original idea but a consolidation of previous technologies.4 In the 1950s various groups of economists (Orcutt, 1957; Clarkson and Simon, 1960; Shubik, 1960) were already working with the idea of digitalising individual and firm behaviours.5 In the 1960s, Thomas Schelling proposed an agent-based model of residential segregation. In the 1980s, the intersection of AI with agent-based models led to ‘multi-agent models’.6
How is an agent-based model built?
ABM starts with the description of a simple behaviour by agents. The agents interact with each other, and the dynamics of the process can be observed at each stage. This is illustrated in Figure 2 below.
Figure 2 Agent-based model construction
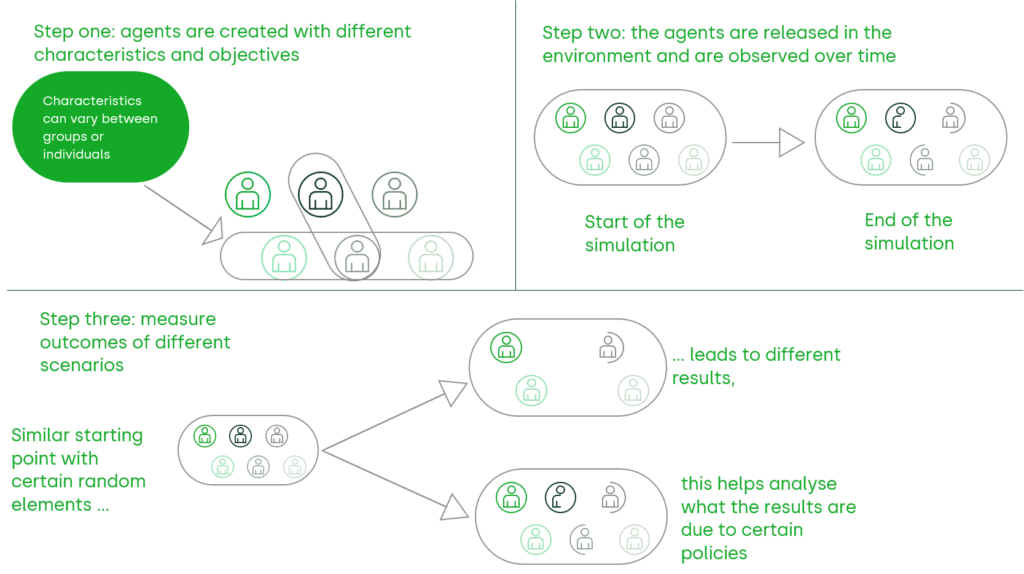
Source: Oxera.
The basic unit of an agent-based model is the agent. A state, a context and the behaviours are the basic elements of each agent, as follows.
- The state is the internal knowledge of the agent (the information that the agent knows but others cannot access). For example, in terms of modelling sales in a shop, the state of a buyer will contain their willingness to pay for goods in the shop.
- The context is all the information shared by the agents. For example, in a retail shop, the context refers to the number of units available for sale, the price(s), etc.
- The behaviours are the logic that an agent will apply to make decisions given a certain set of information. In the retail example above, the behaviours of an agent would include the underlying decision-making process of the buyer about whether and when to buy goods, and how much to buy. This is equivalent to the demand function in traditional economic models, but it will also allow us to model the response of each individual seller. These responses might be dynamic, and allow us to account for relationships between sellers and buyers. In this way, agent-based models allow us to model endogenous relationships that cannot be easily modelled with traditional empirical methods such as econometrics.
Additionally, an agent-based simulation contains global characteristics and steps. The global characteristics are the general inputs to the simulation, such as the number of sellers, buyers and retailers.
Once these variables are defined, the simulation needs to run one step at a time. A step in an ABM simulation executes all the behaviours defined, and then generates new states and contexts for the different agents. Different agents can be at different stages of the same process. For example, one could run a simulation where buyers bid for goods that some sellers offer. As soon as the price that one buyer is willing to pay matches the price at which one seller is willing to sell, the transaction happens, but all the other agents continue bidding. At various points in the simulation, certain buyers may be closing a transaction and others will still be bidding. This approach is more flexible and may be more realistic than the approach used in traditional economic models, where the bids and the processing of the bids are usually assumed to be synchronous.
Applications in economics
ABM has been less prevalent in the economics literature than in other fields. Doyne Farmer and Foley (2009) raised awareness about the need for new tools in economic analysis and proposed the use of ABM.7 Examples of applications in the domain are the study in Gode and Sunder (1993), and the Peitz, Rady and Trepper (2015) experiment.8
Recently, detailed models that attempt to solve real-world economic problems have been presented. For example, Baptista et al. (2016) created an agent-based model of the UK housing market to investigate how macroprudential policies affect housing market indicators.9 They were able to address the heterogeneity of buyers in this market by using ABM to model the individual behaviour and interactions of first-time purchasers, homeowners, buy-to-let investors and renters from the bottom up, and then observe the resulting dynamics in the property and loan markets. The model was calibrated using a wide range of data from household surveys and housing market data sources. The results suggested that an increase in the size of the buy-to-let sector could amplify house price cycles and increase house price volatility. This is due to a larger proportion of buyers and sellers making decisions based on trends and market conditions rather than personal circumstances. In order to test how macroprudential policies affect the housing market, the researchers modelled the introduction of a loan-to-income limit for buy-to-let home owners. The modelled outcomes suggested that this policy would have the effect of attenuating volatility in the house price cycle.
More recently, Heinrich and Gräbner (2019) have criticised the analysis of Rochet and Tirole (2004) in the area of two-sided platforms. As discussed in the box below, Heinrich and Gräbner (2019) propose that the dynamics of two-sided platforms require models that are more transparent in their assumptions, and propose ABM as a solution.10
The application of ABM to study two-sided platforms
In 2004, Rochet and Tirole formalised the analysis of two-sided platforms. The authors created a model to study industries where a platform connects two or more groups of users, such as a marketplace connecting buyers and sellers. Their model takes into account factors that affect the users of a platform, but are not reflected in the prices they pay. These external factors are called ‘usage externalities’ and ‘membership externalities’.
Heinrich and Gräbner (2019) identified limitations to this approach. First, the approach is unable to consider asymmetry in the interactions between buyers and sellers. Second, it requires an assumption that customers have perfect information. Third, the model transforms all per-customer costs and benefits into a per-transaction amount even though some of them do not vary per transaction. Fourth, the negative fees, sometimes offered to attract customers in the early stages of a platform’s development, are not considered. Finally, the model assumes the existence of a unique equilibrium.
As a result, Heinrich and Gräbner show that using an ABM approach is preferable in simulating two-sided platforms. While Rochet and Tirole needed strong assumptions to allow for the tractability of their model, Heinrich and Gräbner’s computational approach was able to relax some of them—such as the assumption of rational platform users with perfect information. In the ABM environment constructed by Heinrich and Gräbner, the dynamics of the system become highly path-dependent. This means that the outcomes of the early stages determine the later stages of the simulation, and therefore the existence of a unique equilibrium is unlikely since many different equilibriums might arise for different initial states. Heinrich and Gräbner suggest that the modelling of two-sided markets requires techniques such as ABM that allow complexity without sacrificing the model’s interpretability.
Source: Oxera analysis of Heinrich, T. and Gräbner, C. (2019), ‘Beyond equilibrium: revisiting two-sided markets from an agent-based modelling perspective’, International Journal of Computational Economics and Econometrics, 9:3. Rochet, J.C. and Tirole, J. (2004), ‘Two-Sided Markets: An Overview’, Institut d’Economie Industrielle working paper.
In 2020, following the same motivation, the ‘AI Economist’ was developed by Zheng et al. (2020).11 The AI Economist uses a combination of ABM and reinforcement learning to explore the impact of taxation in a simple economy. The authors propose a two-level deep reinforcement learning approach to learn dynamic tax policies in dynamic economies. This approach allows for an effective trade-off between economic equality and productivity. Zheng et al. (2020) found that the use of AI-driven tax policies improved this trade-off by 16% over baseline policies, implying that distributional aims of taxation could be achieved while having a less negative impact on the behaviour of those facing higher taxes. This approach does not require knowledge of economic theory or the estimation of the tax elasticity of labour by the agents, making it a valuable tool for governments to consider in designing and testing economic policies.
Why is ABM relevant in solving real-world problems?
ABM has the potential to provide a better approach for solving everyday problems faced by firms and individuals. This is because it allows for greater heterogeneity across individuals, more realistic timing of decisions and transactions, simpler ways to model endogeneity, and the observation of emerging behaviours.
In terms of endogenous relationships, by defining behaviours, ABM can account for bilateral relationships. This can help to disentangle the classic chicken-and-egg paradox observed in many economic problems. For example, when studying the relationship between the profits that firms make in a certain region and the number of firms present—a so-called price concentration analysis—the direction of causality can be complex. Firms with fewer local competitors may be able to make higher profits, but at the same time, the lure of high profits may encourage new firms to enter an area. ABM can help to disentangle these relationships by simulating interactions between firms in a bottom-up way and observing the evolution of the market.
In terms of observing emerging behaviours, starting from simple rules allows for the exploration and identification of unexpected patterns. For example, in a model of platform simulation, starting with a definition of the adoption behaviour(s) of each agent, ABM identifies emerging patterns such as situations in which the winner takes all, or an emergent platform takes over an incumbent.
Additionally, ABM’s ability to consider heterogeneity and non-sequential decision-making processes further enhances its applicability. For instance, when modelling the adoption of a digital platform, ABM can account for factors such as individual preferences, social influences and the propensity to adopt new technologies for each agent, resulting in a more realistic representation of the market. Moreover, in the same context, ABM can consider the lag between an individual joining the platform and their friends doing it later, leading to a more accurate representation of network externalities and platform growth.
Overall, the use of ABM in real-world problems has the potential to provide a more accurate and comprehensive understanding of economic systems and decision-making processes.
Limitations of agent-based modelling
As discussed, ABM can improve economic analysis by creating a tool to explore potential scenarios and the paths not taken (the counterfactuals). But every tool has its limitations, and ABM is no exception.
First, current agent-based models rely on the modeller’s understanding of the reality that they are trying to analyse. In other words, they are reliant on the assumptions and the rules of the system that has been created. In that sense, it is sometimes difficult to justify why agent-based models would be better than theoretical models. For example, even though one could model different types of demand function (behaviour) for the same good, this would be limited to a set number of options usually displayed in the economics literature, such as linear demand and isoelastic demand.
Second, the number of steps in the process can sometimes be more important than the actual behaviours modelled. This brings uncertainty for the modeller about how many steps and how many different scenarios should be run. In addition, the computational power and resources of the modeller may compromise their ability to make the model realistic.
Third, constructing useful simulations is time-consuming. Modellers usually start from scratch, which typically means that they either need to rely on simplifying assumptions that make the model less realistic, or spend a lot of time trying to understand and model a particular part of the reality. Over time, this hurdle may be overcome via standardisation: in the future it is possible that simulations could be constructed from existing elements or building blocks that have previously been developed by others.
Finally, for models to produce useful results, there must be a specific critical mass of agents. In other words, models based on a sample of customers may not lead to a realistic output. This is because, in order for realistic emerging behaviours to arise, the model needs to be as close as possible to reality. When simulating users in a platform, or cars in a traffic jam, the number of agents is one of the main factors. In contexts with little data or low computational resources, this is a disadvantage of ABM relative to statistical tools.
Is agent-based modelling the solution to challenges in economic analysis?
Taking into account both its advantages and its disadvantages, ABM shows a promising capacity to overcome some of the challenges faced by economists. This will depend on the ability to build new simulations on top of existing ones, and the standardisation of modelling to overcome the limitations described in this article. Nevertheless, the use of ABM can be advantageous in at least three circumstances: (i) examining policy changes where the link is endogenous; (ii) determining who will profit and who will lose out when a market structure is changed; and (iii) recognising the effects of a shock on the market’s distribution of outcomes.
1 This is the example of the simple economy on which the ‘AI Economist’ developed by Salesforce AI has been tested, as described in this Google Colab file. Zheng, S., Trott, A., Srinivasa, S., Naik, N., Gruesbeck, M., Parkes, D.C. and Socher, R. (2020), ‘The AI Economist: Improving Equality and Productivity with AI-Driven Tax Policies’, arXiv preprint arXiv:2004.13332.
2 Flow simulation is a way of using computers and mathematical models to predict how fluids will behave in different conditions. It is often used to design and optimise systems that involve the flow of fluids, such as pipes and engines. A mathematical model is used to represent the physical properties of the fluid and the geometry of the system, which is then used to solve equations that describe the flow of the fluid. This allows engineers to predict aspects such as pressure, velocity and temperature, and design more efficient systems.
3 Bonabeau, E. (2002), ‘Agent-based modeling: Methods and techniques for simulating human systems’, Proceedings of the National Academy of Sciences, 99:3, pp. 7280–7287.
4 Axtell, R.L. and Doyne Farmer, J. (2022), ‘Agent-Based Modeling in Economics and Finance: Past, Present, and Future’, INET Oxford Working Papers 2022-10, Institute for New Economic Thinking at the Oxford Martin School, University of Oxford.
5 Orcutt, G.H. (1957), ‘A New Type of Socio-Economic System’, The Review of Economics and Statistics, 39:2, pp. 116–123. Clarkson, G.P.E. and Simon, H.A. (1960), ‘Simulation of Individual and Group Behavior’, The American Economic Review, 50: 5, pp. 920–932. Shubik, M. (1960), ‘Simulation of the Industry and the Firm’, The American Economic Review, 50:5, December, pp. 908–919.
6 Schelling, T.C. (1969), ‘Models of Segregation’, The American Economic Review, 59:2, May, pp. 488–493.
7 Doyne Farmer, J. and Foley, D.K. (2009), ‘The Economy Needs Agent-Based Modeling’, Nature, 460:7256, pp. 685–6.
8 Gode, D.K. and Sunder, S. (1993), ‘Allocative Efficiency of Markets with Zero-Intelligence Traders: Market as a Partial Substitute for Individual Rationality’, Journal of Political Economy, 101:1, February. Peitz, M., Rady, S. and Trepper, P. (2015), ‘Experimentation in Two-Sided Markets’, Available at SSRN, May. Peitz, Rady and Trepper (2015) made use of computer programs that followed simple rules in an auction. In parallel, they conducted the same auction with human traders. As a result, they could compare the two results to extract the characteristics of the market.
9 Baptista, R., Doyne Farmer, J., Hinterschweiger, M., Low, K., Tang, D. and Uluc, A. (2016), ‘Macroprudential Policy in an Agent-Based Model of the UK Housing Market’, Bank of England Working Paper No. 619, October, available at SSRN: https://ssrn.com/abstract=2850414.
10 Heinrich, T. and Gräbner, C. (2019), ‘Beyond equilibrium: revisiting two-sided markets from an agent-based modelling perspective’, International Journal of Computational Economics and Econometrics, 9:3. Rochet, J.C. and Tirole, J. (2004), ‘Two-Sided Markets: An Overview’, Institut d’Economie Industrielle working paper.
11 Zheng, S., Trott, A., Srinivasa, S., Naik, N., Gruesbeck, M., Parkes, D.C. and Socher, R. (2020), ‘The AI Economist: Improving Equality and Productivity with AI-Driven Tax Policies’, arXiv preprint arXiv:2004.13332. An interactive example of how this model can be used to optimise policies to limit COVID-19 infections without disrupting the economy can be found at https://www.salesforceairesearch.com/covid19_sim/index.html.
Related
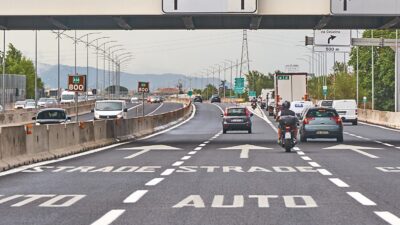
The 2023 annual law on the market and competition: new developments for motorway concessions in Italy
With the 2023 annual law on the market and competition (Legge annuale per il mercato e la concorrenza 2023), the Italian government introduced several innovations across various sectors, including motorway concessions. Specifically, as regards the latter, the provisions reflect the objectives of greater transparency and competition when awarding motorway concessions,… Read More
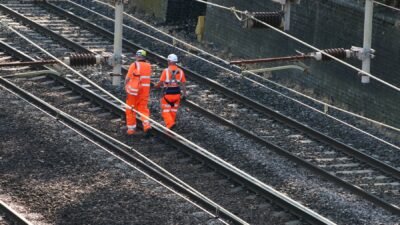
Switching tracks: the regulatory implications of Great British Railways—part 2
In this two-part series, we delve into the regulatory implications of rail reform. This reform will bring significant changes to the industry’s structure, including the nationalisation of private passenger train operations and the creation of Great British Railways (GBR)—a vertically integrated body that will manage both track and operations for… Read More