If data is so valuable, how much should you pay to access it?
In the second of a series of articles about digital markets,1 we turn to focus on data access. Governments around the world have proposed that digital firms be required to provide access to some of their data. This is not unlike initiatives for open data across other industries, from banking to the public sector. If data access is going to be mandated, how can you value the data that a business holds, and set fair and reasonable charges for access to it? Economic tools that analyse the cost of creating the data, and the benefits derived from it, provide critical insight into this question.
Governments around the world are proposing that digital firms be required to provide access to some of their data. These obligations can range from one-off access to real-time data sharing through APIs, and cover not only data for commercial purposes but personal data as well. For example, the EU’s proposed Digital Markets Act (DMA) envisages firms gaining access to data on how end users engage with the products or services provided by them, and search engines providing access to some of the underlying ‘click and query’ data to competitors. Similar recommendations have been made by the UK Digital Markets Taskforce. This is part of a wider regulatory trend for opening up access to data, which started in banking and is spreading to other sectors.
But what is data? In what way is it similar to or different from other assets? A number of articles have described data as the new oil or gold.2 Data is neither of these; but in the right circumstances, some data can be very valuable.
What makes data different (and not so different)?
Figure 1 Main data characteristics
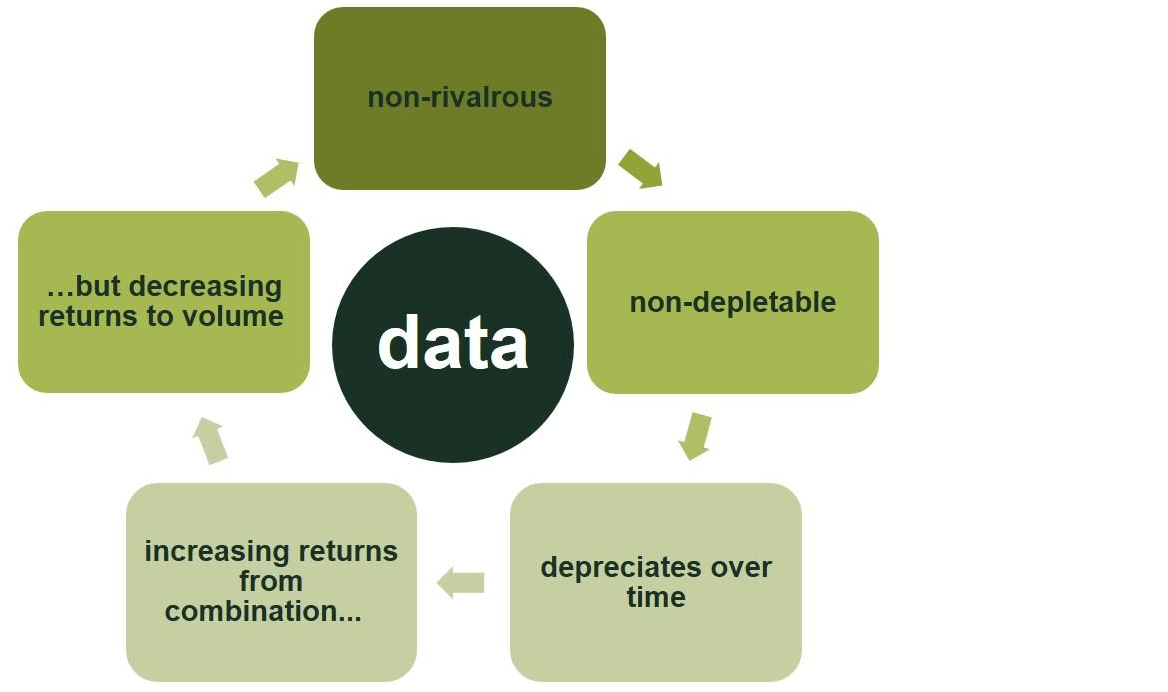
First, data is non-rivalrous. This means that if one firm uses a piece of data, another person can also use that data at the same time for a different purpose. Physical assets are almost always rivalrous in some way: if I have a barrel of oil, you cannot have that same barrel.
Second, data is non-depletable. Essentially, it does not run out—I can use the same piece of data again and again for analysis without using it up or wearing it out. In contrast, the barrel of oil is gone once it has been used to create plastics or burned for energy.
Third, data depreciates over time. Quite how much and how fast it depreciates depends on the data in question. For example, your name, gender and age data depreciate relatively slowly.3 In contrast, data concerning your browsing history, location or the contents of your messages tend to lose their value very quickly. In this respect, data can be similar to physical assets: the barrel of oil does not depreciate quickly, and so can be used now or stored for later use; a fresh cheese in contrast ‘depreciates’ quite quickly.
Fourth, there tend to be increasing returns from the combination of data. For example, supply chains can be fully optimised by bringing together information on the volume and location of products, real-time availability of transport, demand at destination, and storage space. Knowing only one of these factors can improve the management of a supply chain, but knowing all of these factors multiplies the benefits.
Fifth, there tend to be decreasing returns to the volume of data. Training an algorithm requires data. The first few thousand observations are of great value in terms of calibrating the algorithm. Similarly, the next few thousand are also of great value, albeit slightly less so. Eventually, further tranches of data add relatively little to the improvement of the algorithm. An often-cited example of this phenomenon is the Netflix film recommendation algorithm: after a few tens of thousands of data points, the algorithm is almost as accurate as when it is calibrated with a few million.4
Beyond these general characteristics of data, it is important to remember that data is not a homogeneous good since zeros and ones recorded in a database are not equally valuable. Data is context-dependent, and this has a crucial impact on its value.
The current state of the regulatory debate
The debate can be broken down into three layers:
- First, should there be data access? And if so, for what data?
- Second, if there should be data access, what is the process of sharing? How frequently should the data access occur, and will the access be static or dynamic?
- Third, should there be a price for access, and if so, at what level?
There is plenty of debate about the first two questions, and it is far from certain whether and to what extent the various proposals will make the final cut. In this article, we look forward and focus on the third theme: what economic tools and methods exist to value data and put a price on it?
Giving access to data sounds simple—however, data has a cost of acquisition, and sharing that data with others may reduce the value to the owner and reduce their incentive to invest in collecting data in the first place.
Economic regulation has a long history in estimating what the right access price should be. Experience from other sectors tells us that the right access price for data depends on competitive dynamics and the policy objective of providing such access.
If a certain piece of data is vital to offering a service and can only be obtained from one place, then the cost of access might be set at incremental or marginal cost. However, marginal cost pricing provides limited reward for past successful investments and data can often be obtained from different sources—not least through the development of a new innovative service.
In contrast, if the policy goal is greater innovation and investment in data collection, then an access price will have to take into account the ‘value to the owner’ principle, which compensates the access-giver for the risk of investing in the asset and loss of exclusivity.
While the debate on the price for access is commonly addressed in many regulated industries, it has not yet been a major part of the debate in digital markets. It is possible that certain categories of data in digital markets will be provided for free while others will have a price tag associated with them. The EU’s proposals for the DMA, published in December 2020, explicitly envisages that firms can gain access free of charge to real-time data ‘provided for or generated in the context of the use of the relevant core platform services by those business users and the end users engaging with the products or services provided by those business users’.5
The EU proposals for the DMA also envisage that access to search data will be on a FRAND (fair, reasonable and non-discriminatory) basis. A similar recommendation for FRAND access terms comes from the UK Digital Markets Taskforce. But how might we determine what the access price should be?
There is extensive theoretical discussion and volumes of practical experience over the past two decades devoted to determining access prices in telecoms; and further textbooks and cases discuss and describe how FRAND pricing can be calculated for access to intellectual property (IP).6 While the nature of data is unlike that of telecoms infrastructure, and while data may or may not be protected by IP rights, the determination of price for data access is linked to the value of the data itself. Like many other assets, this valuation will be based on the use and/or production of the data.
Relevant questions therefore include: what are the current and potential uses of the data, and what is the data worth to the different (current and potential) users? Are there other types of wider value being generated by use of such data? What is the cost associated with the data?
How to value data—the framework
There are essentially four groups of valuation methods available for the valuation of data, as summarised in Figure 2 below:
- cost-based;
- benchmark/market-based;
- income-based;
- externalities-based.
Given the specific circumstances of the data and the objectives of the party valuing the data, one or more of these methods would be appropriate.
Cost-based approaches, as the name suggests, involve examining the costs associated with creating, storing, processing and sharing the data. For example, purely cost-based approaches (e.g. based on historic marginal costs) work best when the concern or policy objective is about accessing an essential facility that cannot be replicated, and where innovation or incentivising future investment is unlikely.
Benchmark or market-based approaches involve looking at the prices at which data is traded between willing buyers and willing sellers. For example, when the concern is about the fairness of a price, market-based approaches can compare the activity of similar providers or the fees charged for similar assets.
Income-based approaches look to the revenues derived from using the data. For example, the revenues from targeted advertising associated with a particular set of data give a sense of its value. They can also be used when there are concerns about how the economic value of the ‘pie’ is being distributed among the different parties involved since income-based approaches can be provide a bottom-up estimation of future revenues according to each business activity employing that data.
Finally, externalities-based approaches look at the broader impact of the data—for example, the benefits gained by society or users from the data. When the prime objective is to maximise the value to society of the availability of data—for example, when making available data held by a government agency—externalities-based approaches may be the most appropriate.
Often, policymakers or the valuers of data are trading off a number of policy or business objectives, in which case a mix of approaches may be required to arrive at an appropriate value.
The diagram also sets out the basis for the counterfactual—a description of an alternative state of the world—against which one is valuing the data, the sources of information that can be used to undertake the necessary calculations, and the techniques that economists use to undertake the valuation itself.
Figure 2 Data valuation framework
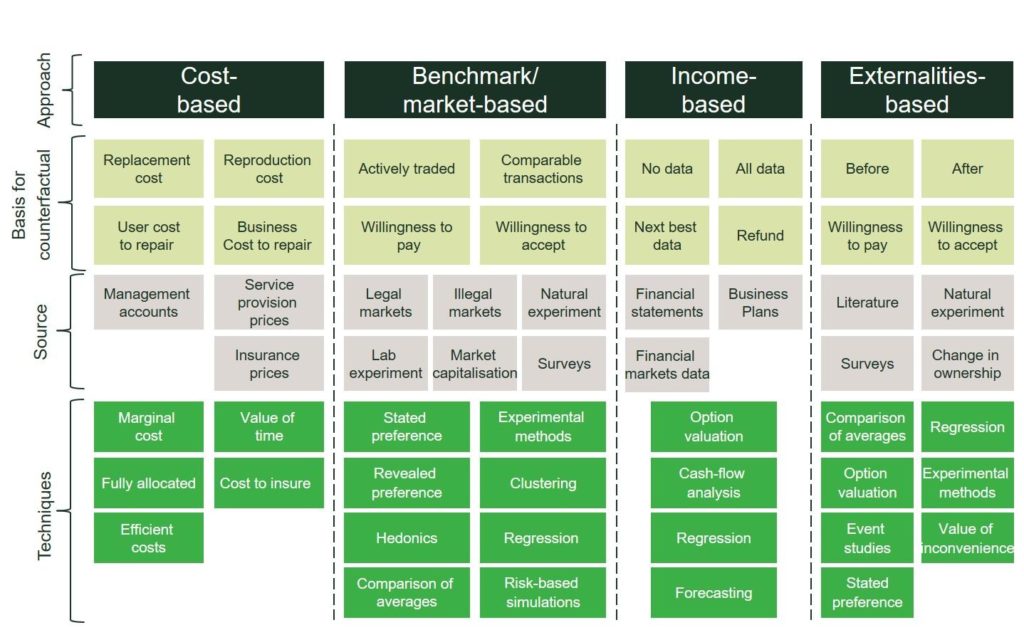
How to value data—examples
As illustrated in the figures above, the economic toolkit provides a wide range of techniques to help quantify each approach based on the appropriate counterfactual and the sources of information available. The illustrative examples provided above are not exhaustive and can be used under more than one valuation method. In the next examples, we show how some of these techniques can lead to a quantification of the value of data.
Stock exchange data
One example of the commercialisation of data is in equity trading markets, where stock exchanges often charge users for access to data (e.g. quotes and prices) generated by their platform. Here, trading data is the outcome of a price formation process, which is a joint product with a trade execution (i.e. it is not possible to generate one without the other). At the same time, regulatory rules can require that delayed data (e.g. more than 15 minutes old) is made available to end-users for free.
A cost-based approach that only considers the costs of producing and disseminating the data may not provide an accurate value assessment, as it does not take into account the joint fixed costs associated with delivering price formation and trade execution—essentially, it is expensive to run an exchange infrastructure. An income-based approach using a cash-flow analysis could compare the proportion of stock exchange revenues attributable to market data with trade execution revenue, as well as with revenues generated by a typical broker or fund manager.7
The value of data can also be determined through a benchmark approach when there are other similar datasets traded. Techniques such as the comparison of averages or regression analysis can isolate the value of particular characteristics—volume, quality, coverage, and the like—of previously traded data to inform the value of another dataset. A similar type of analysis is often used in the valuation of IP.
Atmospheric data
In situations where there are no active data trading markets, one can turn to surveys to provide a guide to the willingness to pay of buyers. For example, in an exercise to value data collected by the British Atmospheric Data Centre, Beagrie and Houghton (2013) estimated this value by using willingness to pay from a survey.8 Because the centre made the data available for free, they designed a survey where respondents shared information on their willingness to pay if they had to pay for access and then multiplied this value by the number of users, which yielded an annual valuation of £5.2m. Using a similar survey technique, the study also estimated the seller’s willingness to accept, which was measured again using a survey of users asking them to consider what rate they would demand if they were deciding the access fee; again this value was then multiplied by the number of users, yielding a value of around £16m.
The difference between the two estimates is consistent with the endowment bias concept–where the owner of an asset assigns it a higher value than when it does not own the asset–and potential financial constraints. This difference highlights the importance of addressing and reducing respondents’ biases through survey design. A potential solution to survey biases is the use of experimental methods in a controlled environment in a lab or through the use of surveys designed for conjoint analysis.
News content and data
Australia is in the process of demanding that digital media companies—notably Google and Facebook—negotiate a usage fee with providers of news content, which can be thought of as a form of real-time data. The News Media and Digital Platforms Mandatory Bargaining Code results from series of consultations from the Australian Competition and Consumer Commission (ACCC).9 If the parties cannot agree a fee, then a binding arbitration process can be triggered. In this arbitration, several of the factors discussed above determine the appropriate access fee, including: the benefits that the content of the news media provides to the digital platform’s service (e.g. advertising); the benefits that the news media firm gains from being on the platform (e.g. additional traffic); and the costs to the news media firm of producing the content.
Google has set out that it considers the benefits that it gains from news in Australia to be quite small: Google does not run ads on Google News, so arguably the direct benefit could be thought to be zero. There may be indirect benefits, but again Google suggests these are small: the firm estimates that it received around AU$10m of revenue from clicks on ads of news-related queries; however, news-related queries make up only around 1% of search queries fielded by the firm.10
The media company Nine—owner of titles such as the Sydney Morning Herald—has annual costs of around AU$450m in its digital and publishing division. How much of these costs one might seek to allocate to an access fee might, for example, be determined by the volume of traffic on platform news feeds relative to traffic on the media companies websites as part of a cost-orientated benchmarking analysis.11
Data access price—a vital piece of the puzzle
There is momentum behind the idea that digital firms should provide access to some of their data in certain circumstances. There remains much to debate about when such access should be granted, precisely which data access will be provided, and the associated technical challenges of making data access a reality.
An important matter that must be addressed concerns the price that should be paid for the access—historically and in other sectors, access prices are not zero. It seems likely that any access price will be related in some way to the value of the data being accessed (more valuable data will therefore command a higher access price) and to the policy objective being pursued by the access obligation. Economic tools that analyse the cost of creating and making the data available, and the benefits derived from it, provide critical insight into this question.
1 The last article in the series is Oxera (2021), ‘Move fast and analyse things: sensible regulation for digital markets’, January.
2 New York Times, (2018), ‘As Facebook Raised a Privacy Wall, It Carved an Opening for Tech Giants’, 18 December;
The Economist (2017), ‘The world’s most valuable resource is no longer oil, but data’, 6 May; CEO Today (2018), ‘Is data the new gold?’, April.
3 Oxera (2018), ‘Consumer data in online markets’, Agenda, August.
4 Amatriain, X. (2014), ‘10 lessons learned from building machine learning systems’, Slideshare.net; Varian, H. (2015), ‘Is there a data barrier to entry?’, Google, slide 18.
5 European Commission (2020), ‘Proposal for a Regulation of the European Parliament and of the Council on contestable and fair markets in the digital sector (Digital Markets Act)’, 15 December, Article 6.i.
6 Oxera (2008), ‘Untangling FRAND: what price intellectual property?’, Agenda, February; Oxera (2017), ‘The right price for intellectual property rights: the debate continues’, Agenda, October.
7 Oxera (2020), ‘What’s the data on equity trading market data? Taking stock of the debate’, Agenda, February.
8 Beagrie, N. and Houghton, J. (2013), ‘The Value and Impact of the British Atmospheric Data Centre’.
9 The Treasury Laws Amendment (News Media and Digital Platforms Mandatory Bargaining Code) Bill 2020 was introduced to the Australian parliament on 9 December 2020.
10 Google (2020), ‘A fact-based discussion about news online’, Google Australia Blog, 31 May.
11 Nine (2020), ‘2020 Full Year Results Announcement’, ASX stock market release, Table 3: FY20 costs AU$433m, FY19 costs AU$482m.
Download
Related
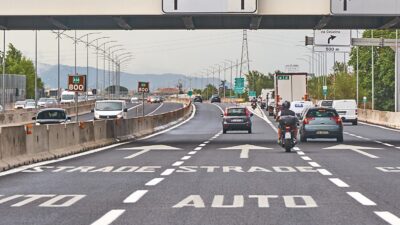
The 2023 annual law on the market and competition: new developments for motorway concessions in Italy
With the 2023 annual law on the market and competition (Legge annuale per il mercato e la concorrenza 2023), the Italian government introduced several innovations across various sectors, including motorway concessions. Specifically, as regards the latter, the provisions reflect the objectives of greater transparency and competition when awarding motorway concessions,… Read More
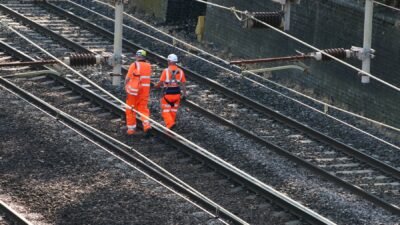
Switching tracks: the regulatory implications of Great British Railways—part 2
In this two-part series, we delve into the regulatory implications of rail reform. This reform will bring significant changes to the industry’s structure, including the nationalisation of private passenger train operations and the creation of Great British Railways (GBR)—a vertically integrated body that will manage both track and operations for… Read More