Using conjoint analysis in merger cases: a competition practitioner’s perspective
If applied correctly, conjoint analysis can be a powerful tool in merger cases, as it can overcome the artificial nature of hypothetical ‘what-if’ questions. The Netherlands Authority for Consumers and Markets (ACM) has used this survey technique in six merger cases to date. Marinus Imthorn, Ron Kemp and Ivo Nobel of the ACM illustrate how it can be used effectively to estimate customer behaviour, and share some lessons from their experience.
Merger control is one of the key tasks of a competition authority. The authority assesses whether a proposed merger might result in customers paying higher prices due to reduced competition. In order to estimate customer demand responses, competition authorities or the merging firms often use surveys to gather evidence by asking hypothetical ‘what-if’ questions, such as ‘would you continue to buy product A if its price increased by 10%’?
These traditional surveys rely on stated preferences of the buyers of a product to determine the likely effects of a ‘Small but Significant Non-transitory Increase in Price’ (SSNIP).1 This raises doubts regarding the predictive validity of the questionnaire approach; buyers might answer hypothetical questions differently from how they would act in practice. As a result, buyers’ price sensitivity is often overstated when they are asked about hypothetical price increases in isolation.
The solution to these problems is to ensure that the decision made by respondents when answering a survey question is as close as possible to the decision made when actually purchasing a good. One way to do this is by using conjoint analysis in the questionnaire. This survey technique has become one of the most widely used quantitative tools in marketing research. Conjoint analysis can help firms to make marketing decisions—for example, about (i) whether to match a competitor’s price increase; (ii) how to price new brands; and (iii) how to set new prices among bundles of existing brands.
How conjoint analysis works
The basic idea behind conjoint analysis is that a product or service can be deconstructed into multiple characteristics, known as attributes. Each attribute can have different levels. A smartphone, for example, can be deconstructed into the attributes and levels listed in Table 1.
Table 1 An attribute list
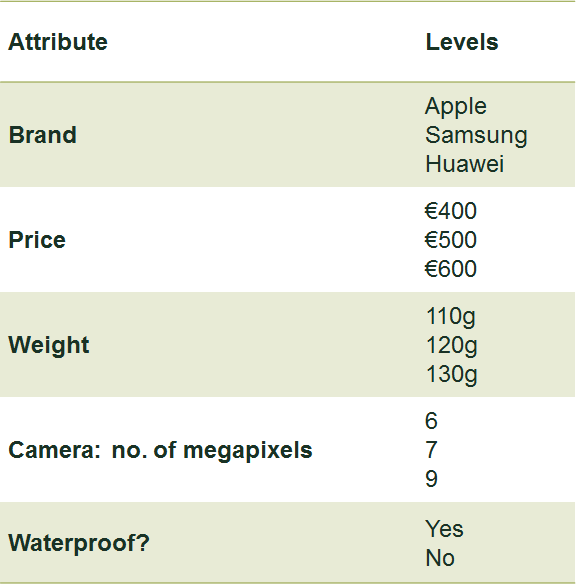
A product profile is a possible combination of attributes and levels, such as the combination ‘Apple’, ‘€500’, ‘110g’, ‘7 megapixels’ and ‘not waterproof’. The respondent of a survey is asked to rank or choose between a limited number of random product profiles. This choice task is repeated several times with different product profiles. With this input, the competition practitioner can analyse how respondents value the different attributes and levels, and the relative importance of the different attributes for the average respondent.
This information can help competition practitioners to assess the closeness of competing products based on product characteristics. The competition practitioner can also calculate what fraction of respondents choose a certain hypothetical product profile over other profiles—i.e. the share of preference. If the chosen product profiles reflect the offerings in the relevant market, the preference shares should be in line with the actual market shares, as shown in Table 2.
Table 2 Preference shares for three hypothetical product profiles
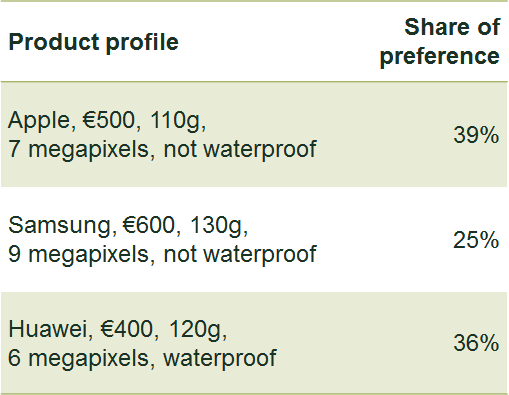
Using these ‘market profiles’ as a base scenario, one can simulate the response of respondents to various changes. For example, it is possible to model how the share of preference for Apple changes if it increases its price to €600. It might be that Apple’s share decreases to 28% and that Samsung and Huawei gain 3 percentage points and 8 percentage points respectively. This information can help competition practitioners to estimate the diversion ratio or the actual loss.2
Application of conjoint analysis in Agrifirm/Cehave (case 6781)
In 2009, the Netherlands Competition Authority (NMa)3 received a notification of a merger between Agrifirm and Cehave, two of the largest cooperatives in the agricultural sector in the Netherlands. The merging firms sell a large number of products to agricultural firms, such as mixed feed for animals and crop-protection products. In the Phase 1 investigation, we concluded that no significant impediment of effective competition was likely for any of these markets, except for one: the market for the sale of artificial fertilisers to agricultural firms. In Phase 2 we investigated this market in more detail.
Thousands of agricultural firms use artificial fertiliser in the Netherlands. In order to make representative statements about this population, the NMa conducted a large-scale survey. Around 1,600 agricultural firms answered the online questionnaire, a response rate of around 9.4%. The questionnaire included a conjoint analysis which served two purposes: (i) to identify the most important factors for customers in the buying process of artificial fertilisers; and (ii) to investigate the response of customers to various price changes.
Conjoint design
We constructed a choice task that closely mimicked the actual purchasing situation of agricultural firms. We chose the choice-based conjoint method because customers tend to buy all their artificial fertiliser from only one supplier, and because the number of relevant attributes is relatively limited. Each respondent was shown three options, as shown in Table 3, and asked to choose their preferred one. The choice task was repeated 13 times with different levels assigned to key attributes in order to gather sufficient information from the respondent.
Table 3 A choice task in the Agrifirm/Cehave case
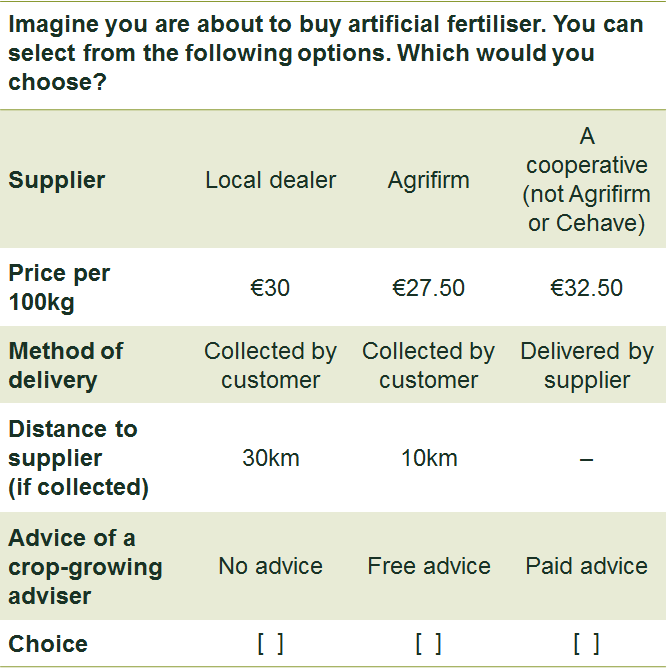
We deliberately chose the attribute level ‘a cooperative (not Agrifirm or Cehave)’ to make it clear to the respondent that we meant a cooperative other than one of the merging firms. We had learned from this potential design error in a previous case. The price attribute ranged from €27.50 to €35 per 100kg.
Relative importance of the attributes
Based on the information obtained, the relative importance of the different attributes was calculated. For the average respondent, the method of delivery (37%) and the price of the artificial fertiliser (28%) were regarded as relatively important in the buying process. The distance to the supplier (18%), the specific supplier (10%) and advice (7%), on the other hand, were relatively unimportant factors.
Post-merger simulations
We specified the base scenario for the simulations after the survey was completed. When specifying this base scenario, based on information from the merging parties, we faced a couple of problems. First, the prices of the common types of artificial fertiliser did not differ significantly between suppliers. Second, suppliers of artificial fertilisers delivered most of their sales to the customer. Only a (decreasing) fraction of the artificial fertilisers was still collected by agricultural firms themselves.4 Finally, most suppliers incorporate the price of advice into the price of artificial fertiliser. Therefore, based on pre-merger market conditions, our base scenario assumed that all suppliers offered the same product: €27.50 per 100kg, delivered by the supplier, and free advice. In effect, therefore, the simulations were able to estimate only the trade-off between the attributes ‘supplier’ and ‘price’. In future cases, we determined the conjoint design and base scenario as early as possible.
We performed simulations similar to the SSNIP test: how would customers react if Agrifirm and/or Cehave raised its prices by 5% or 10%? For example, how would respondents react if only Agrifirm raised its price from €27.50 per 100kg to €28.88 per 100kg (+5%)? The results are shown in Figure 1.
Figure 1 Results of the merger simulations: share of preference after price changes
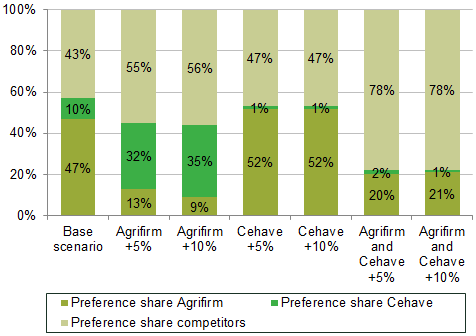
The column on the left (the current situation) represents the shares of preference for Agrifirm and Cehave in the base scenario. Thus, pre-merger, around 57% of the respondents preferred to buy artificial fertiliser from Agrifirm or Cehave. Based on actual sales data, the market shares of the merging firms were found to be similar. The other columns show the shares of preference for various potential price changes by Agrifirm and/or Cehave.
The results give insight into diversion ratios, which are relevant for measuring the closeness of competition between the merging parties pre-merger, and therefore the likely impact of the merger. For example, Cehave would have gained 22 of the 34 percentage points of preference share that Agrifirm lost. This corresponds to a diversion ratio of 65%. The diversion ratio to Agrifirm if Cehave raised its price was 56%. This suggests that Agrifirm and Cehave are relatively close competitors. Finally, the columns on the right show that the combined loss of share if both merging firms raised their prices was relatively high.
Furthermore, the results suggest that agricultural firms are relatively price-sensitive. A small price increase leads to a relatively large drop in the share of preference. For example, if Agrifirm raised its price by 5%, the share of preference would drop from 47% to 13%. Thus, Agrifirm would lose 34 percentage points preference share, or 72% of its customers. The estimated critical loss (i.e. the maximum amount of sales that it could afford to lose before the price rise was rendered unprofitable) was substantially lower than the actual loss. Therefore it is not likely to that Agrifirm would be able to profitably raise its price.
Results from conjoint analysis: an important source of evidence to clear the merger
The results of the conjoint analysis—in particular the simulations—were an important source of evidence in the Phase 2 decision that the merging firms could not profitably raise their prices by 5–10% after the merger. In consideration of this factor, combined with other indications that customers are relatively price-sensitive, and the fact that alternative suppliers had sufficient residual capacity, the NMa approved the merger without restrictions.
Lessons learned
Empirical research is a constant learning experience, and applying conjoint analysis is no exception to this. Three of the lessons that we have learned when using conjoint analysis are discussed below.5
Start early
The conjoint design can be complex and laborious, and it is important to consider it during the early phases of an investigation. It is crucial to understand the real-life choices of customers. In the Phase 1 investigation, the buyers’ selection criteria should be identified, as well as what the decision looks like (purchase of one product at a time, or the use of several suppliers, with one or two preferred suppliers supplying most of the products, etc.). This information can also be used to specify the base scenario. All these issues will influence the design of the conjoint analysis.
Be transparent
Several decisions have to be made during the design phase of the conjoint analysis. Maintaining an accurate record of the choices made contributes to the verifiability of the results. For example, competition practitioners should keep track of the different attributes considered and why certain of these were not part of the final choice tasks, how the data was analysed, and which econometric techniques were used to generate the results. This allows the competition authority or the merging firms to replicate and verify the results.
Test, test, test
As in all survey research, testing of the conjoint design is very important. The test should be conducted with a sample of the actual respondents. During this phase, it will become clear whether the respondents understand the choice task and whether it represents a realistic buying situation.
Concluding remarks
Conjoint analysis can be used in a variety of settings. We have used it for products as well as for services, both for business-to-business and for business-to-consumer markets. By simulating how customers might react to changes in current products (in terms of price or other attributes), conjoint analysis allows competition practitioners to analyse product profiles that vary across multiple attributes. In this way, it mimics as closely as possible the real-life choices of consumers or other buyers when purchasing products.
As with economic techniques in general, the results derived from the conjoint analysis need to be complemented with other types of empirical evidence in order to make an informed decision about the merger in question. For example, it is necessary to assess potential product repositioning, the threat of entry by new firms, and the degree of buyer power.
Marinus Imthorn, Ron Kemp and Ivo Nobel
The views in this article are those of the authors, and do not necessarily reflect those of the ACM. For more information, see Authority for Consumers and Markets (2016), ‘Using Conjoint Analysis in Merger Control: a competition practitioner’s perspective’, ACM Working Paper no. 2, 25 April.
1 SSNIP is a technical term used by competition practitioners as a way of defining relevant markets.
2 The ‘diversion ratio’ represents the proportion of Apple’s sales that would divert to Samsung and Huawei as a result of its increase in price.
3 The Netherlands Competition Authority, the Netherlands Consumer Authority, and the Netherlands Independent Post and Telecommunications Authority (OPTA) joined forces in 2013 to create the ACM.
4 Thus, the attribute ‘distance to supplier’ is irrelevant for most agricultural firms. We tested, using conjoint analysis, whether collecting artificial fertilisers constituted a separate relevant market, but concluded that this was not the case.
5 Our working paper describes five other lessons learned: Authority for Consumers and Markets (2016), ‘Using Conjoint Analysis in Merger Control: a competition practitioner’s perspective’, ACM Working Paper no. 2, 25 April.
Download
Contact
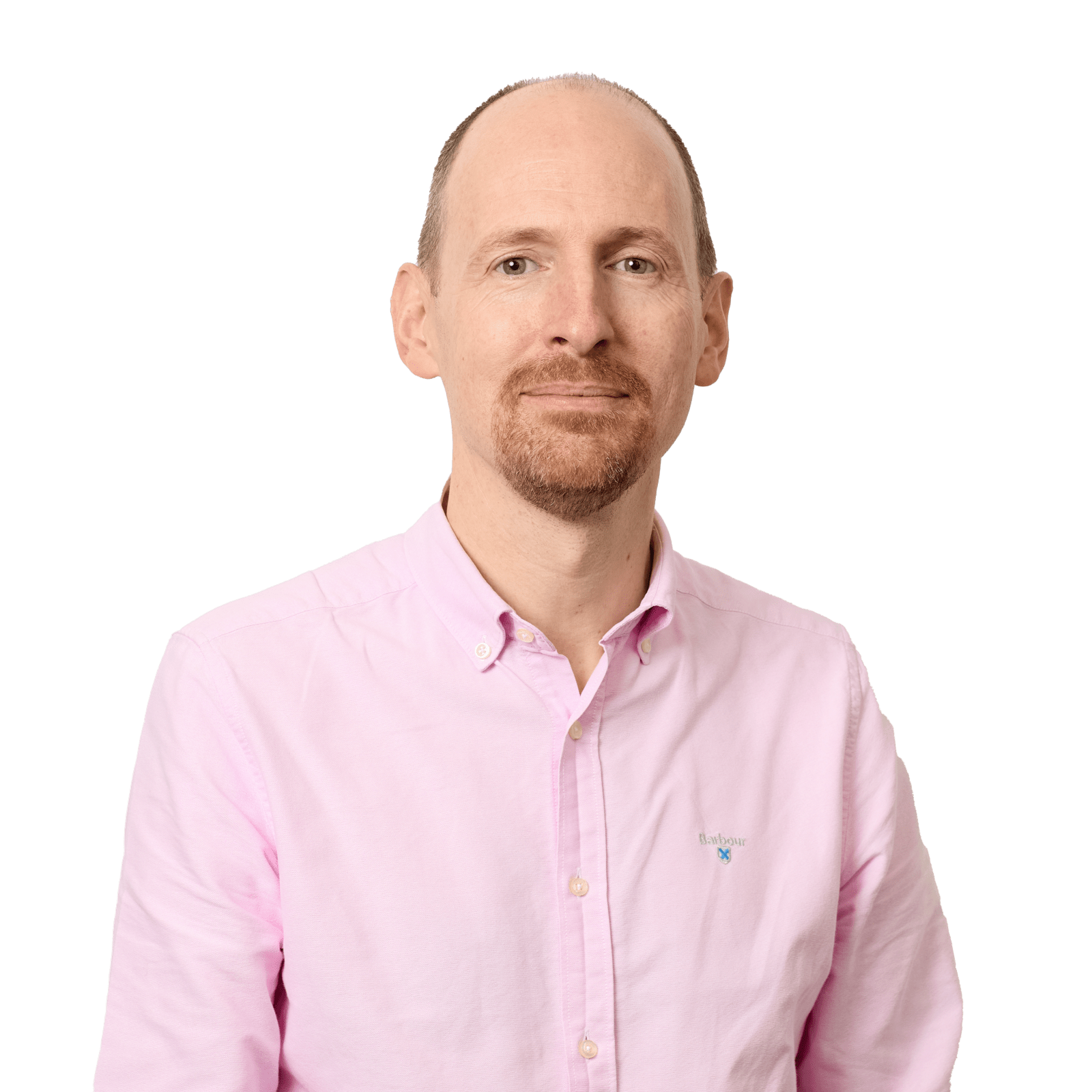
Matthew Johnson
PartnerGuest authors
Marinus Imthorn
Ron Kemp
Ivo Nobel
Related
Download
Related
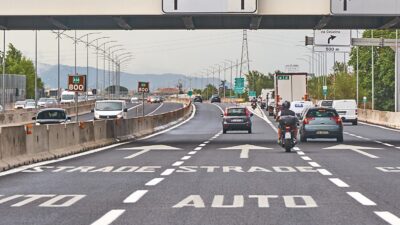
The 2023 annual law on the market and competition: new developments for motorway concessions in Italy
With the 2023 annual law on the market and competition (Legge annuale per il mercato e la concorrenza 2023), the Italian government introduced several innovations across various sectors, including motorway concessions. Specifically, as regards the latter, the provisions reflect the objectives of greater transparency and competition when awarding motorway concessions,… Read More
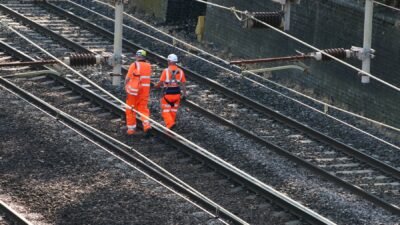
Switching tracks: the regulatory implications of Great British Railways—part 2
In this two-part series, we delve into the regulatory implications of rail reform. This reform will bring significant changes to the industry’s structure, including the nationalisation of private passenger train operations and the creation of Great British Railways (GBR)—a vertically integrated body that will manage both track and operations for… Read More